This project aims to finance seven (7) researchers: a post-doctoral researcher and a team of six (6) PhD candi- dates. They have been organized along (3) phases, analysis, collaboration, and learning. These phases cut across six research topics (one for each PhD candidate). (See image below)
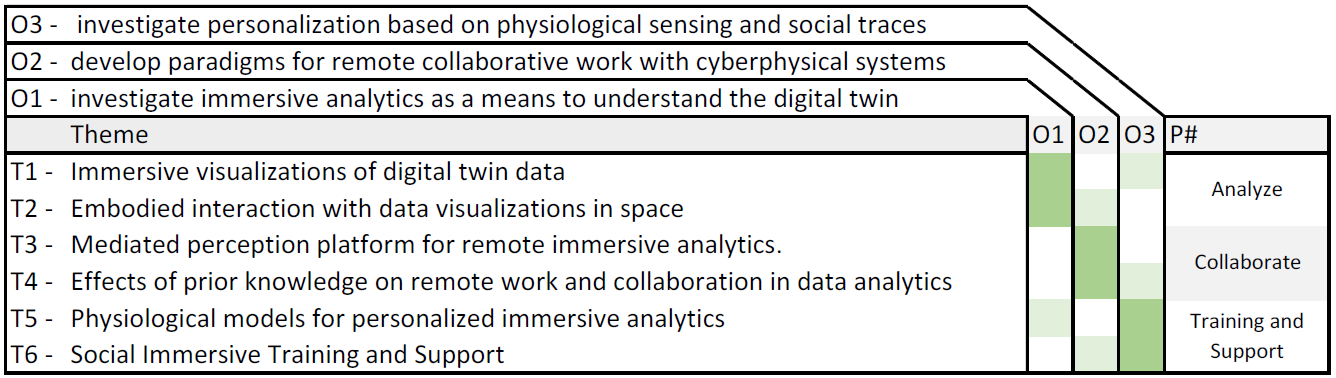
T1- Immersive Interaction with Digital Twin: T1 aims to investigate and advance interactions with the digital twin, including analytics methods and with historic data. Along these lines, we investigate the mental model that the user gains of the digital twin through interaction. This cognitive model will be used to propose novel para- digms to interact with analytics processes, including the manipulation and comparison of the situation presented by the data at various timesteps in the past (from historic data) or future if simulated data are available.
T2- Embodied interaction with data visualizations in space: T2 is intended to investigate the organization of space to deploy various forms of data views and how the user motion and action in space should influence the type of presentation. Data views can be created in 2D or 3D. They can be anchored in space around an object, attached to planar surfaces, or laid out around the user. We investigate various forms of presentation and or- ganization, aiming to make immersive analytics an extension of intuitive motion.
T3- Mediated perception for remote immersive analytics: For a remote user to perform analytics as if being present at the location where the data originates, it is necessary to capture the location and convey it with enough fidelity to the user’s senses. T3 is about research and development of a “mediated perception platform” to deliver first person immersive experience to remote participants, for example using a semi-autonomous robot.
T4- Effects of prior knowledge on experiencing remote work and collaboration in data analytics: We investigate how prior knowledge in the domain affects experience of and performance in remote and collaborative data analytics. Knowing the effect and relative importance of prior knowledge allows 1) taking informed decisions about whether to initiate collaboration in specific circumstances, 2) choosing additional participants for collabo- ration, and 3) taking countermeasures, such as providing upfront information or training.
T5- Physiological models for personalized immersive analytics: We investigate the effects that manifest them- selves in the user during the immersive experience. We will collect physiological data with sensors, incl. eye- tracking, heartrate, galvanic skin response, electro encephalogram, electromyography, etc. We will model the effects on human response and propose personalization and/or optimizations for the immersive experience.
T6- Social immersive training and support: This topic aims at investigating methods to exploit knowledge gained while a user performs the immersive experience. To do so, activity traces will be logged including the user actions in the virtual environment. We will investigate the use of activity traces in two ways: for personal- ized recommendations, and to create workflows for tutorials.