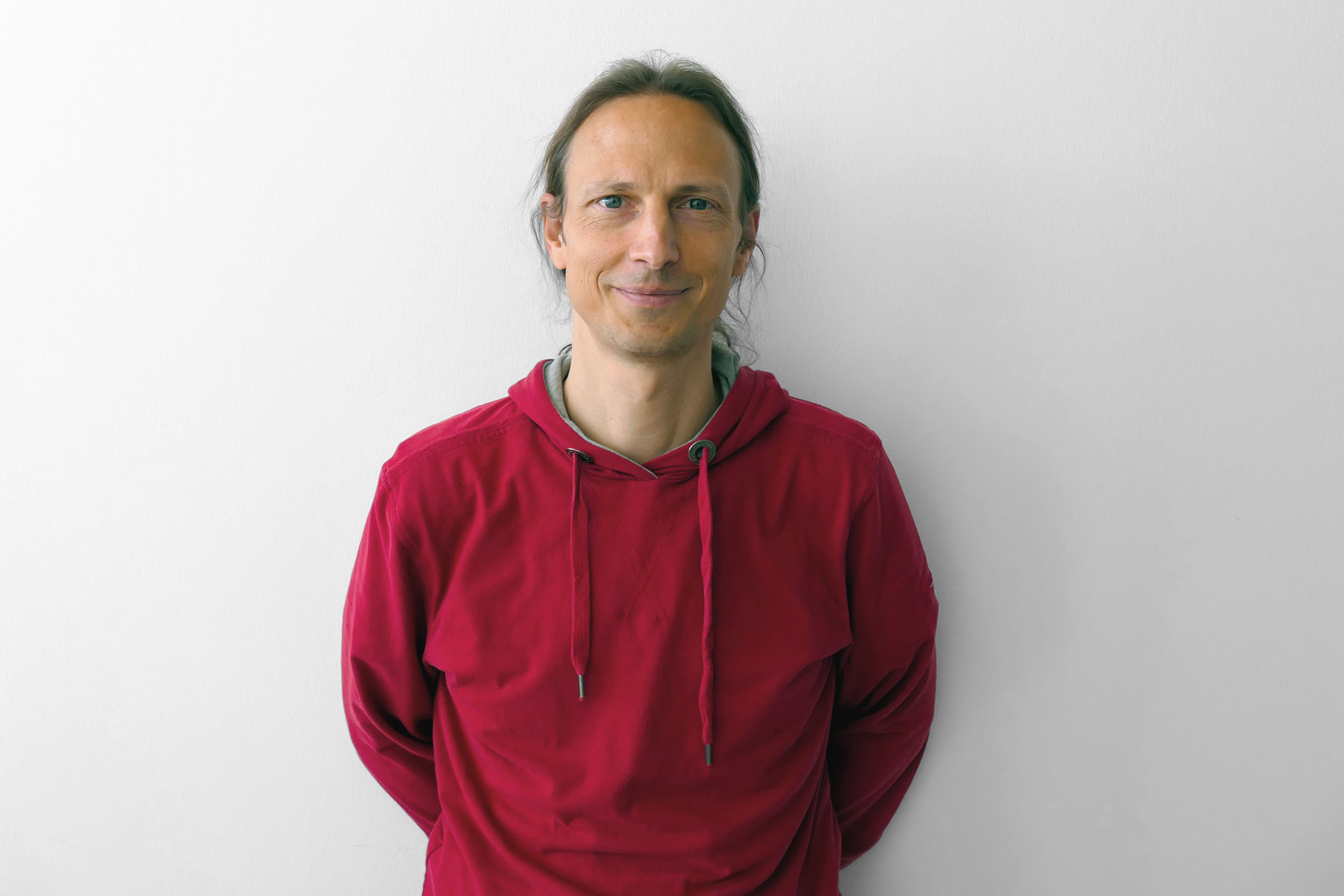
How would you explain the term “knowledge discovery” to a child?
It’s about deducing knowledge from data. This specific knowledge must be beneficial and new, i.e., something unknown. It is needed wherever data exists. For example, in medicine to improve diagnoses, in industry to make production processes more efficient or to recognize patterns in data, such as people’s shopping behavior.
What research questions are you currently working on?
I am working on NLP, which stands for Natural Language Processing, and the analysis of textual data. I am currently interested in the question of causality. Data by itself doesn’t reveal anything about causal relationships, only correlations are evident. In most cases, this is sufficient for predictions, but not if you want to make decisions based on data. It’s about recognizing the right causalities and linking data intelligently to bring success for companies.
Can you be a little more specific?
In our day-to-day work, data science deals with very particular questions. For example, a customer learns that there are quality deviations in his product and wants to know more about the causes. In an industrial context, however, the results of calculations are often difficult to assess – you need expert knowledge to this end. When working with bank data, for example, we require background knowledge of the processes, e.g., which transactions occur in case of credit card fraud. Causality helps us identify which additional information we need, and we specifically can request the domain knowledge from the customer. This enables us to not only make predictions, but to better assess the impact of decisions.
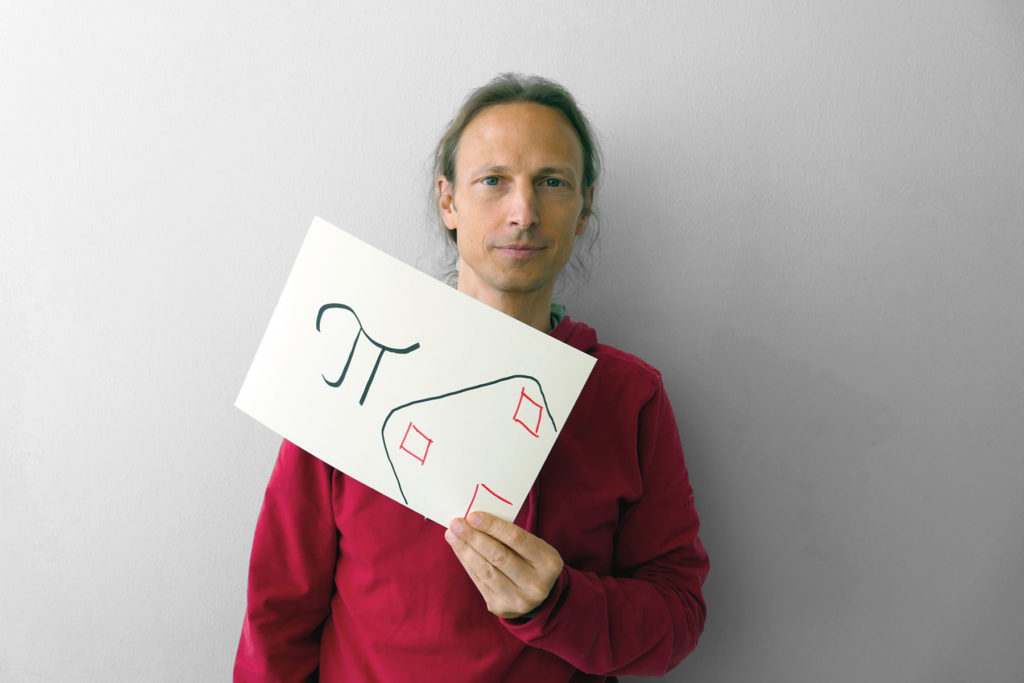
BIAS
People make all kinds of assumptions without even thinking about them. Even science is not immune to it. The charm of working with machines lies in the fact that machines are better than humans when it comes to estimating probabilities based on explicit basic assumptions.
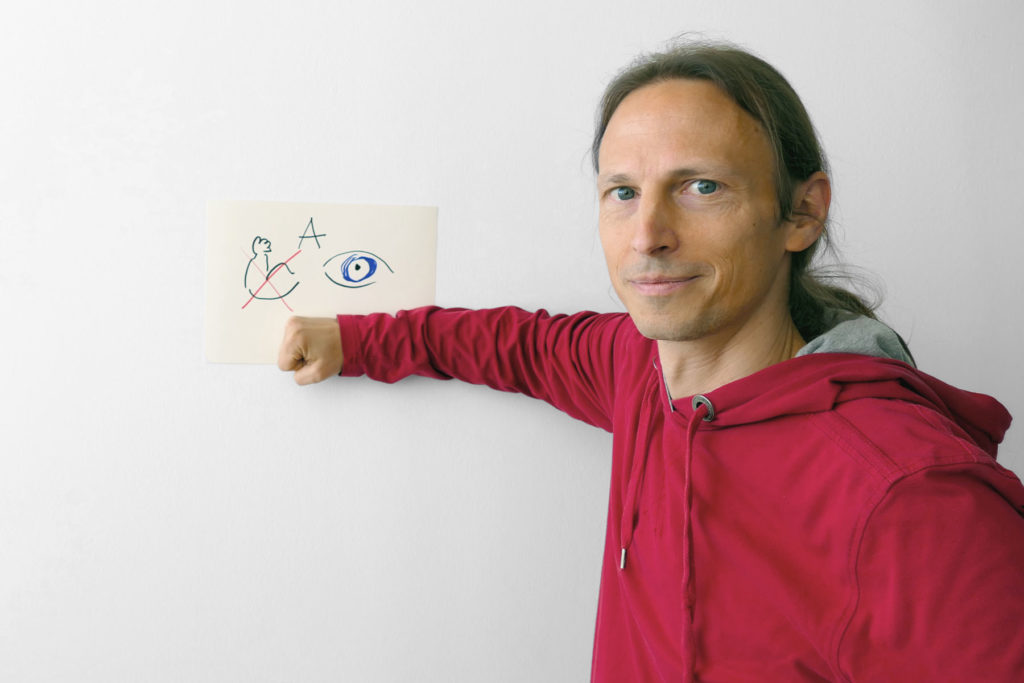
Weak AI
People are afraid of artificial intelligence replacing them at work or that they might become smarter than humans. While in fact, it’s about AI supporting them in their work by processing large amounts of data and recognizing patterns. Predictions are only as good as data. This is referred to as "weak AI."
What is NLP all about?
The volume of text written by humans is continuously increasing. These texts are unstructured or semi-structured at the most, which means not usable by the machine instantaneously. We must preprocess and extract them, which is a lot of work. At this point I am also interested in fundamental questions. In my doctoral thesis, I investigated whether it is possible to recognize an author directly by his or her writing style. Humans are surprisingly bad at that. It would prove helpful in forensics, for example, to classify blackmail letters or in medicine to recognize psychological conditions. The methods aren’t good enough just yet to assign texts to individual people. But it already works quite well with aggregated data of multiple people. For example, Facebook posts could be used to determine people’s age based on their writing style.
What captivates you about your subject area?
Working in my area is awesome. The technical progress in recent years has been enormous. We are currently in a ‘Storm and Stress’ kind of time, where a lot is tried out but still to some extent lacking comprehension. Most of the time an algorithm works great, but we don’t know why it works so well. That’s why we need explainable AI, so users can understand how a program’s AI works and how to evaluate the results.
What’s your way of approaching research questions?
I always work on solutions, entirely independent of the technology. In the end to the customer it doesn’t matter whether it’s a 100-layer deep learning algorithm or which exact technology was used. He is interested in having his problem solved. Nor is privacy and data protection just a marketing slogan by Know-Center. At least as far as my personal privacy is concerned as I got neither Google, Microsoft nor Apple installed on my cell phone (laughs). What I’m getting at is we’ve got a very small-scale economy in Austria and supply chains are becoming increasingly digitized. Products are digitally accompanied along the supply chain. The question is, what do these digital artifacts look like? What internal company or confidential information is disclosed? Suitable technologies are needed to protect such data.
Do you have a guiding principle that accompanies you?
“Don’t try to solve a problem under pressure.” I always have plenty of ideas – which doesn’t mean they’re good ones – but it reduces some of the stress if you can rely on always coming up with something. Students often put themselves under enormous pressure, which impedes creativity. In research, many times you work on problems which don’t have a solution (yet). That’s pretty much the definition of research.
Would you recommend your students to pursue a research career?
That’s a good question. To good students I would recommend joining Know-Center (laughs). I’m quite serious – a research institution like Know-Center has the advantage of allowing insights into both research and industry. Within the COMET projects that we carry out one can get a general idea on how a big company or a start-up works. That’s very helpful for someone who can’t make up their mind.